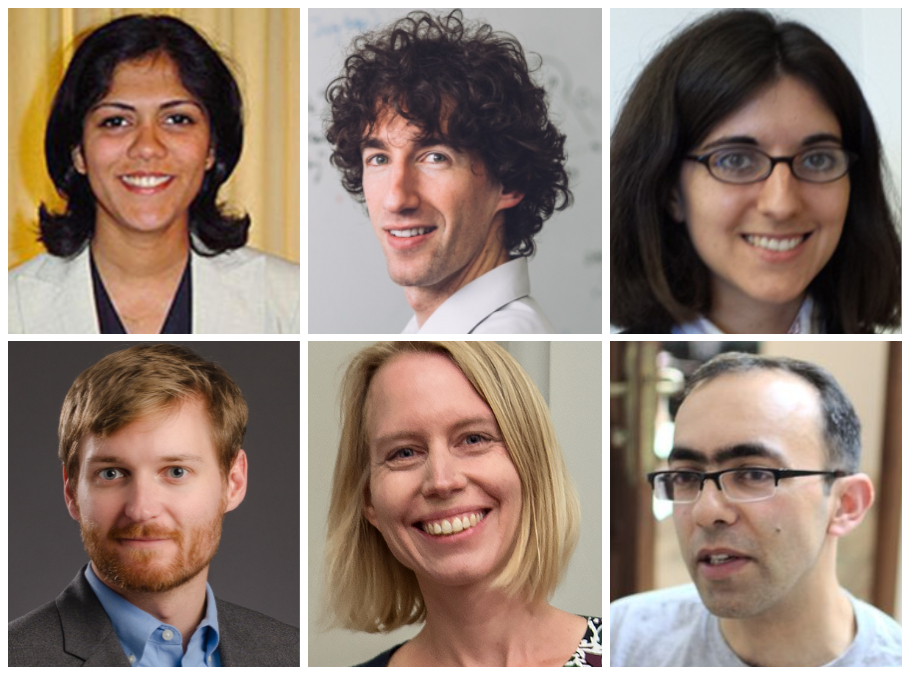
Six IDSS faculty awarded promotions
IDSS is delighted to share that five faculty members have been promoted to the rank of associate professor without tenure, effective July 1, 2019: Guy Bresler and Suvrit Sra (EECS and IDSS core faculty members), Tamara Broderick and Stefanie Jegelka, (EECS faculty and IDSS affiliate faculty members), and Dean Eckles (Sloan faculty and IDSS affiliate faculty member). Additionally, Hamsa Balakrishnan (Aero/Astro faculty and IDSS affiliate faculty member) has been promoted to Full Professor.
Hamsa Balakrishnan received her PhD in Aeronautics and Astronautics from Stanford University in 2006. Prior to joining MIT, she worked at the NASA Ames Research Center. Hamsa joined MIT in 2006 as an Assistant Professor in Aero/Astro, and is associated with the Operations Research Center as well as an affiliate faculty member of IDSS. Currently, she is the Associate Department Head of Aero/Astro and the Director of Transportation@MIT. Her research is in the design, analysis, and implementation of control and optimization algorithms for large-scale cyber-physical infrastructures, with an emphasis on air transportation systems. Her contributions include airport congestion control algorithms, air traffic routing and airspace resource allocation methods, machine learning for weather forecasts and flight delay prediction, and methods to mitigate environmental impacts. She was a recipient of the American Automatic Control Council’s Donald P. Eckman Award in 2014, the AIAA Lawrence Sperry Award in 2012, the inaugural CNA Award for Operational Analysis in 2012, the Kevin Corker Award for Best Paper of ATM-2011, and the NSF CAREER Award in 2008.
Guy Bresler received his PhD in EECS from the University of California at Berkeley in 2012. After a post-doctoral fellowship at MIT, he became assistant professor in EECS and a core faculty member of IDSS in 2015. Guy’s research focuses on high-dimensional statistical inference. His work considers two key factors for an inference or learning task to be possible: (1) informational/statistical complexity (does the data contain enough information for the task to be in principle feasible?), and (2) computational complexity (does the problem have a structure that can be exploited in order to obtain computationally feasible algorithms?). He has made several central contributions to this field. Recent work addressed the question of “complexity” of statistical problems. This work is, in many ways, an “average complexity” analog of the traditional field of worst-case complexity in theoretical computer science. In groundbreaking work, Guy developed a comprehensive theory that maps out a rich web of relations between important statistical problems such as sparse PCA, community detection, bi-clustering, and others, ultimately showing that these problems are at least as hard as the problem of discovering a planted clique in a random graph. In statistics, the planted clique problem is accepted as a computationally intractable problem (within a certain parameter regime), which is analogous to what happens in traditional complexity theory where NP-hard problems are accepted (conjectured) to be intractable.
Guy has taught and contributed to the curricula of multiple EECS classes, including probability theory, machine learning, algorithms for inference, and discrete stochastic processes. He mentors several PhD students; a student of his won the competitive best paper award in the Conference on Learning Theory (COLT). He serves as co-chair of the ML/INFR student admission group in EECS, and organizes external seminars in both LIDS and SDSC.
Tamara Broderick received her PhD from UC Berkeley in 2014 and joined the MIT EECS faculty in January 2015. Tamara works in machine learning and statistics. Her research focuses on Bayesian inference and graphical models — with an emphasis on scalable and nonparametric methods. She has made many significant and foundational contributions to her fields. For instance, her work demonstrates how to summarize data as a pre-processing step before running Bayesian, and other, machine learning methods. Her methods are fast and practical, come with theoretical guarantees, and are already widely used. Her work also demonstrates how to quickly and automatically calculate a local form of sensitivity to assess modeling assumptions in Bayesian analysis. This work simultaneously provides dramatically improved uncertainty quantification for popular Bayesian approximations.
Tamara has received an NSF CAREER Award, a Sloan Research Fellowship, an Army Research Office Young Investigator Award, a Google Faculty Research Award, an International Society for Bayesian Analysis Lifetime Members Junior Research Award, and a Marshall Scholarship. In addition, she received the Jerome H. Saltzer Award for Excellence in Teaching in recognition of her phenomenal teaching ability. She is also a sought-after lecturer, having given more than 100 invited talks, seminars, and tutorials worldwide. Tamara is an affiliate faculty member of IDSS.
Dean Eckles received his PhD in communication from Stanford University in 2012. Before joining MIT, he worked on the Facebook Core Data Science team, with previous work at the Stanford Persuasive Technology Lab, the Nokia Research Center and Yahoo! Research Berkeley. He joined MIT in 2015 as an Assistant Professor of Marketing at MIT Sloan and an affiliate faculty member of IDSS. His substantive research examines people’s interactions with and through communication technologies, especially the ways these technologies mediate, amplify, and direct social influence. Within these areas, he has studied peer effects in networks, the spread of rumors and (mis)information, design of experiments in networks, evaluating high-dimensional methods for observational causal inference, mobile persuasive technologies, self-disclosure and sharing behaviors, multi-armed bandit problems, and persuasion profiling. As this research sometimes requires or benefits from new analytical methods, Dean also works on applied statistics, design of field experiments, and causal inference. Recently, he was the recipient of a 2018 Amazon Research Award, which recognizes and funds outstanding research projects conducted by PhD students or postdocs under the supervision of the faculty member awardee.
Stefanie Jegelka received her PhD in computer science in 2012 from ETH Zurich and the Max Planck Institute for Intelligent Systems. After serving as a postdoctoral researcher at UC Berkeley, she joined MIT EECS as an assistant professor in early 2015. Stefanie’s work span the theory and practice of algorithmic machine learning and optimization. She is considered a leader in submodular optimization, a field that has been extremely important for computer vision and machine learning to accommodate problems with discrete combinatorial structures. Her work combines deep theoretical understanding with practical motivation and efficient implementation, providing algorithms with exceptional practical performance and rigorous theoretical guarantees for several key problems. Her current work also studies negative association in discrete probability and machine learning (which enables diverse selection of data points) and discrete probabilistic inference and Bayesian optimization. In addition, Stefanie has made significant teaching contributions to the department. She developed a graduate course, “Learning with Combinatorial Structure,” that covers models, algorithms, and applications, analyzing how various types of mathematical structures can be used for machine learning. She also co-developed (with Caroline Uhler) a new hands-on data analysis course, “Statistics, Computation, and Applications.” She teaches several other courses, including 6.862 (Applied Machine Learning), 6.437 (Inference and Information), and 6.046 (Design and Analysis of Algorithms).
An active member of the machine learning community, Stefanie has organized several workshops on optimization and combinatorial machine learning, given invited tutorials, and has been on the senior program committee for several conferences, including NIPS, ICML, AISTATS, and UAI. She has received an NSF CAREER Award, a DARPA Young Faculty Award, the German Pattern Recognition Award, and a Sloan Research Fellowship. Stefanie is an affiliate faculty of IDSS.
Suvrit Sra received his PhD in computer science from the University of Texas at Austin in 2007. He was a senior research scientist at the Max Planck Institute for Intelligent Systems and a principal research scientist in LIDS before he joined the EECS faculty in 2018. He is also a core faculty member of IDSS.
Suvrit is a leading researcher in the active area of optimization for machine learning. His research bridges areas such as optimization, matrix theory, geometry, and probability with machine learning. Convex optimization methods, in particular stochastic gradient descent (SGD) and its variants, have become the standard workhorse in this area with much recent progress. Suvrit has made fundamental contributions to both convex and nonconvex optimization for machine learning, including developing variance reduction techniques for nonconvex problems with significant improvement over SGD and finding tractable classes of nonconvex optimization problems by exploring the underlying geometry of the problem and discovering the “hidden convexity” in important problems. More broadly, he is interested in data-driven questions within engineering, science, and health care.
Suvrit has contributed significantly to the department’s educational mission by teaching both graduate and undergraduate courses in machine learning. He is also active in the larger machine learning community. He founded the OPT (Optimization for Machine Learning) series of workshops at the NIPS conference, which he has co-chaired since 2008; he has also edited a popular book with the same title, published by MIT Press in 2011. His work has won several honors at machine learning venues, including the 2011 SIAM Outstanding Paper Prize.