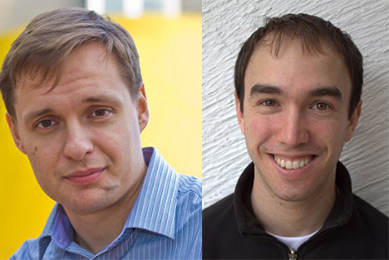
Yury Polyanskiy and David Sontag granted tenure
LIDS and SDSC affiliate faculty member Yury Polyanskiy and SDSC affiliate member David Sontag were two of seven School of Engineering professors whose tenure was announced this week.
Polyanskiy is a theorist who works on information processing systems that arise in communication, control, and learning. He is widely known for his pioneering work on finite blocklength information theory. His work developed fundamental results in non-asymptotic information theory, providing tight lower and upper bounds for the capacity of a given blocklength. He also has important contributions in a broad set of areas including properties of information measures, discrete geometry and combinatorics, and statistical learning theory. The tools and relations he developed for information measures enabled him to settle long-standing conjectures in network information theory and address fundamental questions in control and high-dimensional statistics.
He has taught the undergraduate courses Introduction to EECS (6.02) and the SuperUROP course (6.UAR). He is currently co-developing a new foundation-level class, Introduction to Data Science (6.S077). He also teaches the graduate classes Information Theory (6.441) and Fundamentals of Probability (6.436), and he co-developed a new graduate class, Tools of Discrete Probability (6.265).
“I was delighted to learn of this recognition of Yury’s achievements, while not being the least surprised, as Yury’s intellectual power and seminal contributions are exceptional,” said LIDS director John Tsitsiklis. “His enthusiasm is inspiring and contagious, and it is a privilege to have him with us.”
“As a core faculty member, Yury has been a tremendous presence in IDSS from its start,” added IDSS director Munther Dahleh. “This is a wonderful recognition of his talent and energy.”
David Sontag focuses on research in machine learning and applying machine learning to health care. In machine learning, he focuses on graphical models, which provide a mathematically rigorous and computationally efficient way to represent dependencies between a hidden (latent) structure and observations. His contributions include new highly efficient algorithms for learning, inference, and prediction with graphical models from real world data, and theoretical results in a form of error bounds and correctness proofs that establish a new framework for theoretical analysis of approximate and exact learning and inference in graphical models.
Sontag is a pioneer in applying machine learning expertise to health care, where he has significantly advanced the state of the art in building predictive models from electronic medical records. “David’s work at the interface of machine learning, statistics, and health care adds an extremely important dimension to SDSC,” noted SDSC Director Devavrat Shah. Sontag’s expertise in clinical decision making has led him to build novel formulations of machine-learning problems. The analytical tools and algorithms he develops to solve those problems are advancing machine-learning fundamentals and having an impact beyond health care.
Sontag is also the Hermann L. F. von Helmholtz Career Development Assistant Professor in the Institute for Medical Engineering and Science (IMES) and principal investigator in CSAIL. His honors include an NSF CAREER Award, faculty awards from Google, Facebook, and Adobe, several best-paper awards, and the EECS George M. Sprowls Award for Best PhD Thesis.