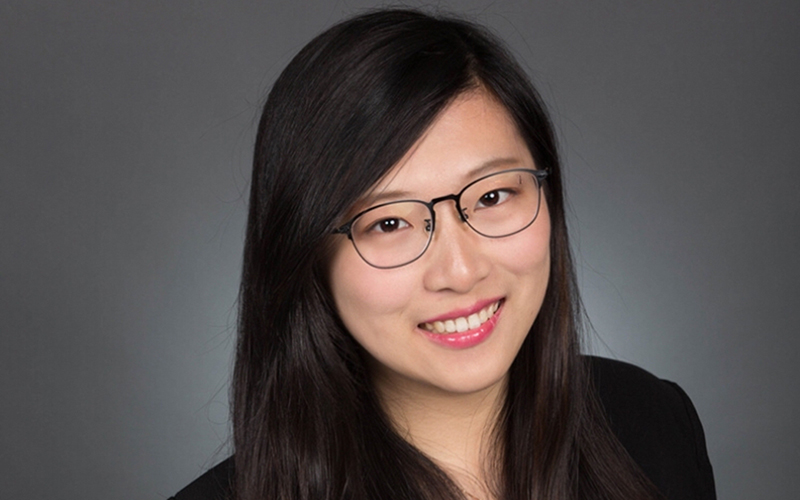
Student profile: Manxi Wu, SES
Manxi Wu is a final year Ph.D. candidate in the Social and Engineering Systems program at the MIT Institute for Data, Systems, and Society. Previously, she obtained B.S. in Applied Math from Peking University (2015) and M.S. in Transportation from MIT (2017).
Her fields of interest are societal networks, game theory, network optimization, and the theory of economic incentives. Her research focuses on the study of platforms for information provision and autonomous services. She develops models for strategic learning, information design, and incentive mechanisms to achieve socially desirable outcomes in socio-technical systems.
Manxi is a recipient of the Hammer Fellowship, UTC Milton Pikarsky Memorial Award, Siebel Scholarship, and an EECS Rising Star.
1. What is the focus of your research? What sort of knowledge and disciplines does it bring together? How will it make an impact?
My research is motivated by the increasingly central role of AI-driven platforms in today’s socio-technical systems and the need to understand their impact on our society. I am interested in studying how platforms and autonomous systems affect the system functionality by re-shaping the information environment and incentives of users and service providers. I also aim to develop guidelines to address societal issues in the design of these complex systems.
My Ph.D. research focuses on transportation networks as the system domain. These networks continue to suffer from inefficiencies and fragility to disruptions, despite the advances in traffic sensing, routing and mobility services. In my work, I take the approach of building models that address the strategic nature of human decision makers, while also accounting for the physical constraints and dynamics of the engineering system. My analysis and results have led to new insights on designing socially-aware navigation services that reduce congestion and infrastructure overuse. I also provided algorithmic design of incentive mechanisms for autonomous transportation systems.
2. Why did you choose to come to IDSS/SES?
I believe the Social & Engineering Systems doctoral program will help me develop technical expertise to study the issues at the intersection of engineering design and economics of smart cities. I find the IDSS weekly seminar course and the monthly Distinguished Seminars particularly helpful because they provide me the opportunity to learn the fundamental research in the relevant areas, as well as expose me to other broader research frontiers.
3. What do you do in your spare time?
The ongoing pandemic has raised fundamentally new questions about the interplay between human mobility patterns both within and across metropolitan areas, and the safe operational capacity of various transport modes (particularly, public transportation and shared mobility). I have been studying these questions in the past few months using the mobility and COVID datasets acquired from New York City and Odisha (an Eastern state in India).
In the study of New York City, I built a panel regression model that evaluates the causal relation between mode-specific mobility patterns and the case growth rate, while accounting for various confounding factors such as test allocation and demographic and socio-economic factors at zip-code levels. I found that while a higher level of general mobility is associated with increased cases, public transit may not contribute to additional case growth during the summer when mandatory masks and social distancing rules were in place. Moreover, my analysis showed that test accessibility in NYC during the pandemic was not equitable across regions with different socio-economic and race distributions, and this inequity was not fully resolved even in the reopening phases in late summer.
Additionally, in the context of Odisha, I developed a networked epidemiological model to study the district-wise COVID growth rates due to the sudden and uncontrolled influx of incoming migrant workers when the India-wide lockdown was lifted in early May 2020. I designed a simple and effective testing strategy to contain the COVID growth rates in a localized manner based on available data on confirmed cases, testing capacity, and inter/intra-district travel.
I find the MIT community inclusive and encouraging. In my spare time, I enjoy hiking and cooking.