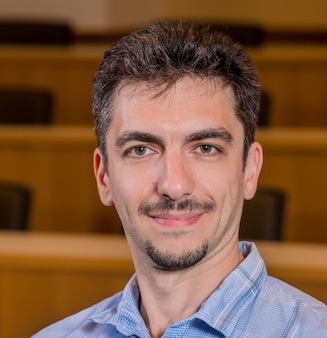
Models of learning from data: a Q&A with IDSS Associate Professor Alexander “Sasha” Rakhlin
Alexander “Sasha” Rakhlin is an Associate Professor in Brain and Cognitive Sciences and IDSS. His research is in machine learning, with an emphasis on statistics and computation. He is interested in formalizing the process of learning, in analyzing learning models, and in deriving and implementing emerging learning methods. A significant thrust of his research is in developing theoretical and algorithmic tools for online prediction, a learning framework where data arrives in a sequential fashion.
Prof. Rakhlin received his bachelor’s degrees in mathematics and computer science from Cornell University, and doctoral degree from MIT. He was a postdoc at UC Berkeley EECS before joining the University of Pennsylvania, where he was an associate professor in the Department of Statistics and co-director of the Penn Research in Machine Learning (PRiML) center. He was also a Visiting Professor in IDSS’s Statistics and Data Science Center (2016). He is a recipient of the NSF CAREER award, IBM Research Best Paper award, Machine Learning Journal award, and COLT Best Paper Award.
What are some of the unique or important aspects of your work?
I’m interested in models of “learning from data.” These range from classical statistical models of parameter estimation to more modern questions in the realm of Statistical Learning, to new models of predicting in an online fashion based on a growing corpus of data. The latter framework, in particular, has been at the center of my research for several reasons. I believe it better describes many of the real-world applications where data arrives in a stream, and I can only imagine that online methods take center stage in near future for the obvious reasons of the ever-increasing amount of data. Second, the online framework allows much weaker assumptions on the data than those employed by classical statistics, hence yielding more robust methods. Last, but not the least, the framework introduces a wide range of new exciting questions and leads to many new beautiful and nontrivial algorithms. Over the last 7 years, we have developed a theoretical foundation of online methods, but there are still many mountains to climb!
What sort of knowledge and disciplines does your work bring together? Are there opportunities to collaborate with other faculty?
My research is centered on statistics and computation. The first aspect refers, roughly speaking, to the size of data required to learn or estimate, while the second is an algorithmic question. As such, my work draws on tools from Probability/Statistics (in particular, Theory of Empirical Processes) and tools from Algorithms/Optimization. Some of the most exciting work, in my opinion, came from observing strong connections between these different fields. In fact, I feel lucky to be working in an area that requires such a diverse set of ideas.
There is no doubt in my mind that statistics and computation should be studied in tandem. IDSS is home to an impressive group of scientists with expertise in these very subjects. I am excited to join the group, and I look forward to many collaborations over the years to come.
How did you get started on this path? What inspires the work that you do?
Since my undergraduate years, I remember being fascinated by the idea that one can make a computer “learn” from data without pre-programming the knowledge. This was the reason I entered the Ph.D. program in the Brain & Cognitive Sciences department at MIT, where I studied approaches to formally quantifying sample complexity of learning methods.
To this date, I’m inspired by the close connection to the real world and the direct applicability of the methods. This is especially true with the line of work on online methods, as insights into statistics and computation immediately yield new efficient prediction strategies that we implement on a computer and try out on data.
What do you like about the MIT community?
I enjoy the intensity, the breadth of topics being discussed, and the depth of knowledge that my new colleagues have. In particular, IDSS is a unique place in this respect. I like the warmth and friendliness of my colleagues, and I am extremely excited to join the group. Oh, and it looks like I will start playing hockey again (thanks to the IDSS team for the invitation).