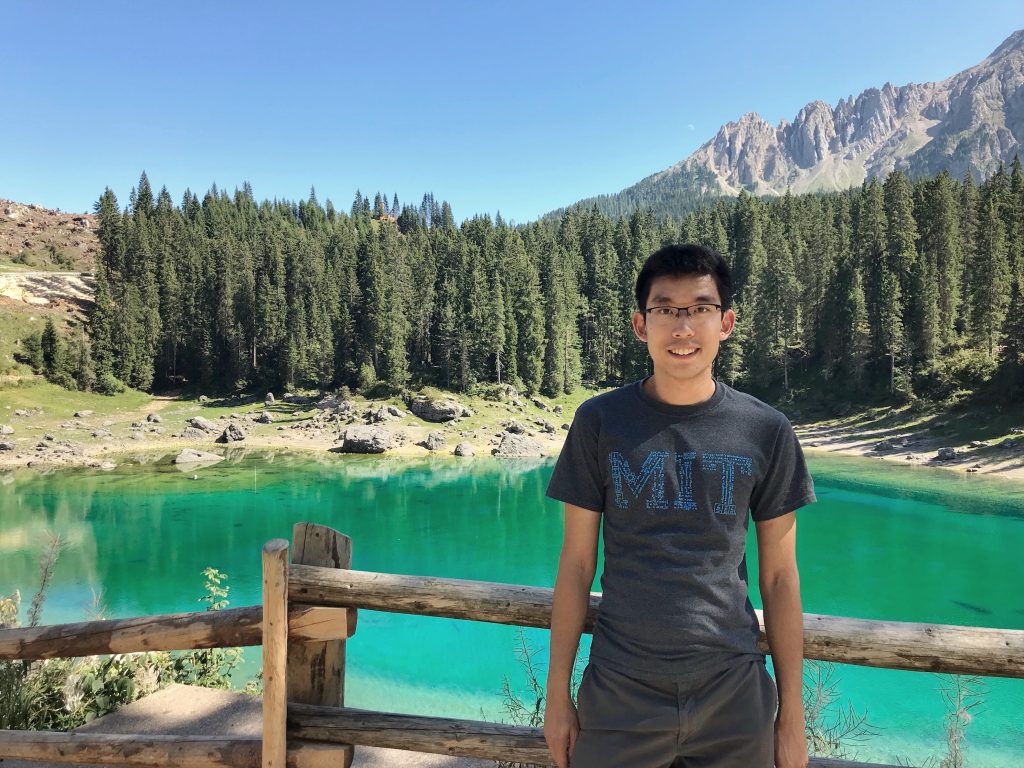
Modeling the impacts of sustainability policies
“Imagine waking up at 8:00am and thinking it’s 8:00pm because it’s so dark.” That’s what Minghao Qiu and millions of people experienced during the ‘Beijing Haze,’ a catastrophic air pollution event in the winter of 2013.
Qiu researches how energy and environmental policies affect air quality and human health as a PhD student in IDSS’s Social and Engineering Systems program (SES). He’s recently co-authored a paper published by Environmental Science & Technology that makes a case for using a data-driven evaluation of past policies’ impact to improve predictions of future policies’ effects.
“The central message we want to deliver is that retrospective analyses of past policies can help make smarter projections of future policies,” says Qiu. By analyzing firm-level energy and pollutant emissions data, Qiu and his co-authors examined the impacts of energy policies in China that were focused on energy-intensive industrial sectors. They sought answers to questions like: Were companies compliant? Which companies achieved or overachieved their goals?
And ultimately, did the policies work?
Policy analyses
Like the Great Smog of London, another pollution event in 1952, the Beijing Haze was both disastrous and predictable, pushing many in China to confront the intersections of pollution, economic growth, energy use, and policy. Qiu was studying environmental science and economics at Peking University in Beijing at the time. To him, the haze was a wake-up call for all of China, and it cemented his resolve to research air pollution, energy, and policy as a complex system.
The retrospective, data-driven approach is called ‘ex post,’ as opposed to ‘ex ante,’ a prospective approach that is more common in research in this field. Qiu and his colleagues argue that comparing ex post to ex ante analysis can help in “going back to the policy to see if it delivered what it promised to do.”
Retrospective analyses are limited by data availability. On top of this, the data that does exist is far from cut and dry. “When you look at real-world data, it is pretty noisy and is driven by many covarying factors,” explains Qiu. “If you see air pollution drop, it could be due to your policy, or it could be that the economy was not growing as fast. It could be weather. It could be something else.”
Qiu identifies two key covarying factors to air pollution change: social and economic factors, and meteorological variability. His work seeks better statistical methods to account for these covarying factors so that the impact of a policy can be better assessed. They use these methods to construct a ‘counterfactual’ of what might have happened if the policy had not been implemented.
“There are a lot of projections, but not many are evaluated,” says Qiu. “There is little retrospective work – we want to fill this gap.”
Social impact
Qiu and his colleagues argue that their method can be applied to other complex problems. “It’s been interesting to think through how data could be used to solve a lot of societal problems, and also inform my research solving environmental problems,” says Qiu.
In the SES program at IDSS, Qiu found himself uniquely positioned within a community that addresses broad societal challenges with rigorous data analysis. The research of his SES peers has helped him gain greater perspective on how environmental issues link with other parts of human society.
“Developing policies to address multiple challenges simultaneously is vitally important for sustainability,” says co-author Noelle Selin, an IDSS professor with a joint appointment in the Department of Earth, Atmospheric, and Planetary Sciences (EAPS) and Qiu’s research advisor. “Research like this is necessary to better understand how actions on air pollution, climate change, and other challenges are connected, and their potential benefits for people near and far, and living now and in the future.”
“Air pollution is localized, climate change is global,” adds Qiu, “We’re all in the same boat with the climate problem.”