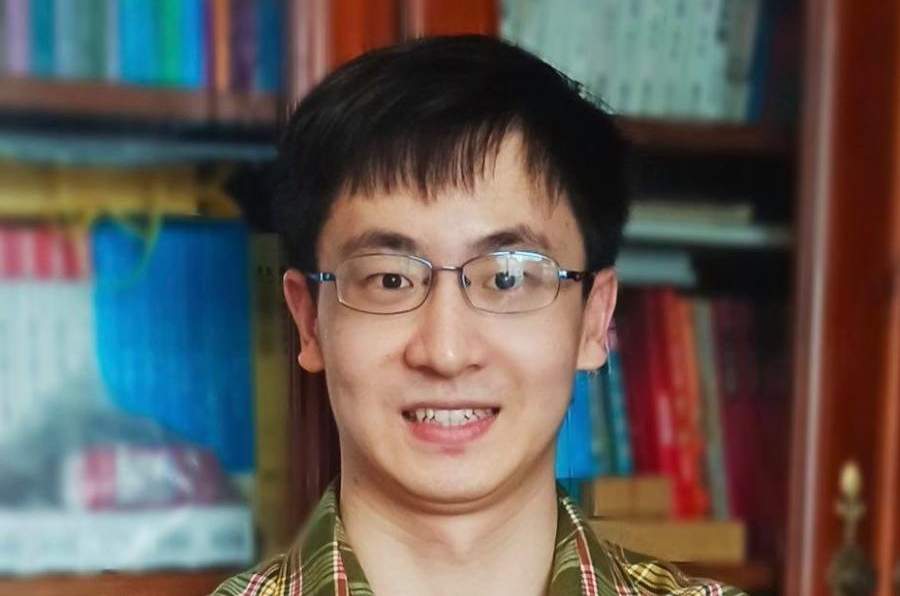
Managing hidden risk in operations
Feng Zhu’s research interests lie broadly in sequential (or online) decision-making, with primary applications to experimentation and supply chain management. Zhu is methodologically interested in understanding how to manage (hidden) risk in modern decision-making environments with uncertainties. Before joining MIT, he majored in Mathematics & Statistics and minored in Economics at Peking University.
What is the “multi-armed bandit” problem, and what new insights does your research bring to it?
The stochastic multi-armed bandit (MAB) problem is a classic framework in sequential decision-making theory. It involves a fixed set of options, or “arms,” each with an unknown probability distribution of rewards. The term “bandit” derives from imagining each option as a slot machine (one-armed bandit) in a casino. Each time one of the arms is “pulled”, a reward is obtained. The objective is to maximize the cumulative reward over a time horizon. The challenge arises because the distribution of rewards for each arm is initially unknown to the decision-maker. The stochastic MAB problem illustrates the trade-off between “exploration” learning the distributions through repeated interactions – and “exploitation” – collecting as much reward as possible. This problem has numerous applications in real-world scenarios where choices must be made on-the-fly with incomplete information, such as dynamic pricing, clinical trials, and financial investment.
Existing approaches to the MAB problem mostly use the metric of efficiency – maximizing the expected cumulative reward, or equivalently minimizing the expected regret, where regret refers to the difference between the cumulative reward obtained by always pulling the best arm and by executing a policy that does not a priori know the reward distributions. The performance of a policy is often characterized through its expected regret’s growing rate. However, if an MAB policy design only focuses on minimizing the expected regret (or efficiency), the design may not have safety against the probability of incurring a large regret – in reality, one may only collect a single realization of the outcome instead of the average case. For example, in clinical trials, while maximizing expected welfare is of primary importance, neglecting to properly account for tail events of incurring a large welfare loss can lead to misleading conclusions on a new drug, hard-to-replicate results, or even significant legal and financial repercussions for drug manufacturers. My work thus tries to answer the following questions: How to achieve (optimal) safety under optimal efficiency? What is the (optimal) trade-off between efficiency and safety?
Our results reveal a delicate and precise balance between efficiency and safety in different scenarios (so-called “instance-dependent” and “worst-case”), and under different knowledge of the experimentation time horizon. In particular, we find that a large class of policies considered in the literature suffer from low safety, meaning the tail probability of incurring a large regret decays very slowly as the policy interacts with the environment more. We propose a new policy design that leads to both optimal efficiency (regret expectation growing at the lowest rate) and high safety (regret tail probability decaying ay the fastest rate). Our policy design has interesting implications to AI – it surprisingly coincides with what was adopted in AlphaGo Monte Carlo Tree Search (Page 7 of the Nature article, the action selection phase). Our theory provides some high-level insights to why their engineered solution is successful and should be advocated in complex decision-making environments.
What other approaches to hidden risk in sequential decision making has your research explored?
An important part of my research tries to address the real-world challenges of managing disruption risk in supply chain operations. I look at how sequential decision-making and data science tools can be adapted to enhance the ability of a supply chain to prepare for, respond to, and recover from disruptions. My ultimate goal is to establish a framework that enables the responsible utilization of supply chain data, fostering alignment with industry partners’ goal and safeguarding daily supply chain operations. I have been working with several large companies (e.g., Accenture, DENSO, Ford) throughout my PhD study to develop risk detection methods (e.g., disruption prediction models) and develop strategies (e.g., inventory control policies) to reduce the vulnerability to disruptions in their supply chains. In Summer 2023, I spent a great time working on inventory simulation and optimization at Ford Motor Company as a supply chain analytics intern.
One particular direction I have been working on is unifying and extending existing risk exposure concepts and proposing new ones to measure supply chain resiliency. A part of my work has been highlighted in Harvard Business Review: Fixing the U.S. Semiconductor Supply Chain, where through working with DENSO we supplement the concept of Time-To-Recover (the time needed for a supplier to recover to normal operations after being disrupted, or TTR) by proposing a new concept called Time-To-Recover-Inventory (or TTRI, the time needed for the whole supply chain to recover after a disrupted supplier gets recovered, such that the inventory level in each node of the supply chain recovers to its pre-disruption level while ensuring all demands are satisfied). The hidden risk of a supplier should be evaluated not only on its TTR, but also on the TTRI associated with its TTR.
However, in practice companies often possess only limited distributional information about supplier disruption profiles and make production planning decisions sequentially and irrevocably as a disruption unfolds. This observation, combined with the series of above-mentioned practical work, leads to a working paper, where we answer the following question: What is the impact of uncertain disruption profiles in supply chain disruptions on pre-disruption risk detection, intra-disruption response coordination, and post-disruption system recovery? Our work highlights that uncertain disruption profiles can significantly alter the risk exposure of suppliers compared to the case when disruption profiles are fully known, and emphasizes the importance of coordinated response among plants. We also demonstrate the advantage of our framework through a comprehensive case study.
Apart from the work mentioned above, I have also explored on other topics including Bayesian online multiple testing and handling non-stationarity in revenue management. I hope my study contributes to a deeper understanding of sequential decision-making though an operational lens, offering new perspectives that bridge theoretical rigor with practical applications.
What do you do outside of your research? How do you connect with the MIT and Cambridge communities?
I started playing the Erhu, a traditional Chinese musical instrument, at the age of six. Throughout my PhD studies, I have been actively involved with the Chinese Music Ensemble. Additionally, I have both organized and participated in Spring Festival Chinese Music Galas in 2023 and 2024.
I have a deep passion for singing at any given opportunity. Since the beginning of 2023, I’ve been a proud member of HAcapella – a group of Asian individuals from the Great Boston Area based in Harvard University who share a fervent enthusiasm for performing popular songs in A cappella styles.