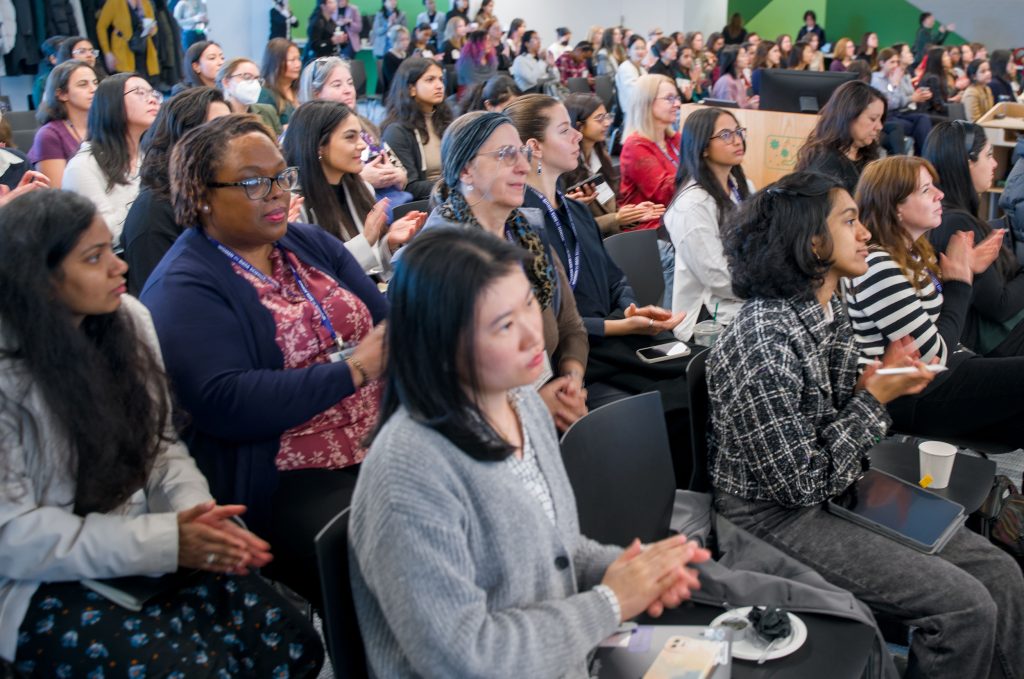
Building a community of practice for women in data science
If your goal is to help a vulnerable group of people, you need information about them. Who are they, where are they, what do they need? But when a population is experiencing violence, even basic data collection can pose a risk.
The ethics of data collection and data analysis was a steady theme throughout the day at the 8th annual Women in Data Science (WiDS) Cambridge conference, where talks by academics and industry leaders explored using data in humanitarian work in conflict zones, and democratizing AI tools so that more people can contribute to data-informed efforts to address societal challenges.
The WiDS Cambridge conference, a collaboration between IDSS, Harvard, and Microsoft, is a local part of the Women in Data Science Worldwide initiative first started at Stanford in 2015. The local conference draws in students and professionals alike for a day of women-led talks.
“A humanitarian crisis is a stochastic environment. There are so many variables,” said Erica Nelson, a panelist who co-directs Humanitarian Geoanalytics Research and Education Programs at the Harvard Humanitarian Initiative. As such, there are countless opportunities for data science to add value to humanitarian work, from evaluating and improving the effectiveness of interventions, to measuring causes and effects, to modeling and predicting outcomes.
On the other hand, “crises create opportunities for opportunists to take advantage of vulnerable populations,” added fellow panelist Daphne Joseph, a Research Fellow at McKinsey.
Talks at WiDS also covered technical topics, including a close look at Bayesian causal frameworks. Poster presenters displayed applications in many domains, and structured networking opportunities were available throughout the day.
“I loved hearing about the projects of the other women at the conference,” said Erin Walk, a grad student in the IDSS Social and Engineering Systems (SES) doctoral program who presented a poster at WiDS. “Data science is such an interesting field because we all work on very different subjects, but there is a shared language of methodology that enables us to speak together meaningfully even across disciplines.”
Community service
Nelson’s humanitarian work is in the field of geoanalytics, which offers something akin to a shared language: a useful framework for uniting disparate data.
“Being in a stochastic space, we have to start to incorporate multidisciplinary, transdisciplinary, and interdisciplinary methodologies, conceptual models, and data sources,” she said. “Geoanalytics is an extraordinarily profound way to do that, because everything exists in time and space, and space and time can be those things that unite all of this multidisciplinary data.”
At the same time, practitioners said that analyzing data without sufficient context will not lead to effective insights or solutions. “Building out conceptual models based upon my assumptions, based upon my experiences … they’re just going to be wrong,” said panelist Rana Hussein, a researcher at the BU Center on Forced Displacement
“We want students to be able to communicate across disciplines, and we want to equip students with an understanding of the limitations of relying solely on data, and to be able to critically examine the data that they’re using,” she added.
“I find it’s so important to not just look at the numbers, but also to compliment them with surveys, research, and interviews … to provide a holistic picture of the needs of people so that you can create the best impact,” agreed Joseph.
“In collaboration with our partners, this is the user-centric design process,” said Nelson. “The communities in which we serve actually are the experts.”
One solution to the many challenges of interdisciplinary, data-informed work in humanitarian spaces, Nelson added, is “building up communities of practice” — like the Women in Data Science Worldwide community — where researchers and practitioners in different areas can share knowledge and learn how to better collaborate.
How sure are you?
In a keynote on the subject of using data to navigate conflict frontlines, Fotini Christia centered the importance of grounding data analytics in a causal inference framework. Christia, a political science professor and Associate Director of IDSS, chairs the SES doctoral program and directs the Sociotechnical Systems Research Center (SSRC). Through her fieldwork in places like Afghanistan, Bosnia, Iraq, and Yemen, Christia has decades of experience in research design that brings the lab to the field, asks divergent sets of research questions, mixes methodologies and data tools, and constantly seeks to validate its findings.
“We are trying to identify causal effects and provide information on underlying mechanisms, while thinking about how to integrate different methods in this process,” she said.
Data collection work can be extensive and, as Christia is quick to point out, expensive. Mixed method designs can exploit natural experiments, argued Christia, who has employed randomized control trials (RCTs), field experiments, lab experiments in the field introducing randomization, and remote data analysis — just to name a few examples.
“So the idea is to combine all these different data sources, while contextualizing your evidence, and asking: what are the underlying mechanisms of the effects?’ she said. “All while validating your data with information from the ground.”
“When we’re talking about causal relationships, we want to know what’s happening under the hood,” said Danish Baker, a data scientist and statistician at Microsoft who gave a plenary talk on causal inference. “How do I understand the causal structure of the data underlying my problem? Considering these things helps us to make solutions more robust, more interpretable, and even more fair.”
Baker showed how the Bayesian inference method in particular serves not only to quantify uncertainty with continuously updated assumptions based on new data, but also to provide an estimate of confidence in those probabilities.
“How sure are you in the recommendation that you’ve provided?” she asked. “We need to think in terms of policy making, of things that could affect nations — of things that can affect women.”
In Bayesian inference, probability distributions are set on parameters, meaning that calculations are influenced by prior assumptions about the structure underlying the data. To Baker, this is all the more reason to operate across disciplines.
“You have to be willing to collaborate, because we are providing prior information for how we think about the data.”
Data literacy
The WiDS community in the Cambridge area is growing, with more events throughout the year such as meetups and datathons. While IDSS provides some leadership and support, many in the WiDS community itself are motivated by a desire to see more data skills in their fields.
“We need more people in different sectors across society who are literate in what AI is, what it may be used for, and when it’s helpful,” said Priya Donti, a panelist on AI democratization and professor of Electrical Engineering and Computer Science (EECS) at MIT.
Donti is the co-founder of Climate Change AI, which strives to bring more computing tools to the climate change research space, including by providing funding for education. “We want to enable more people to contribute to that ecosystem,” she said.
“IDSS co-sponsoring WiDS is part of a commitment to expanding data science education,” asserted Christia. “There is now a growing collection of online programs developed by IDSS faculty under the IDSSx banner. These offer rigorous but flexible upskilling opportunities for learners at a range of skill levels.”
“The annual WiDS conference is a great opportunity for our local online learners and our residential students to network,” she added.
For Walk, who presented a poster about her SES dissertation research into polarization and segregation in online content viewership, the conference fostered new connections for future research.
“I met people from other departments at MIT with whom I could share similar experiences and strategies for navigating the complexities of this field — whether that’s through career advice or sharing datasets,” she said.