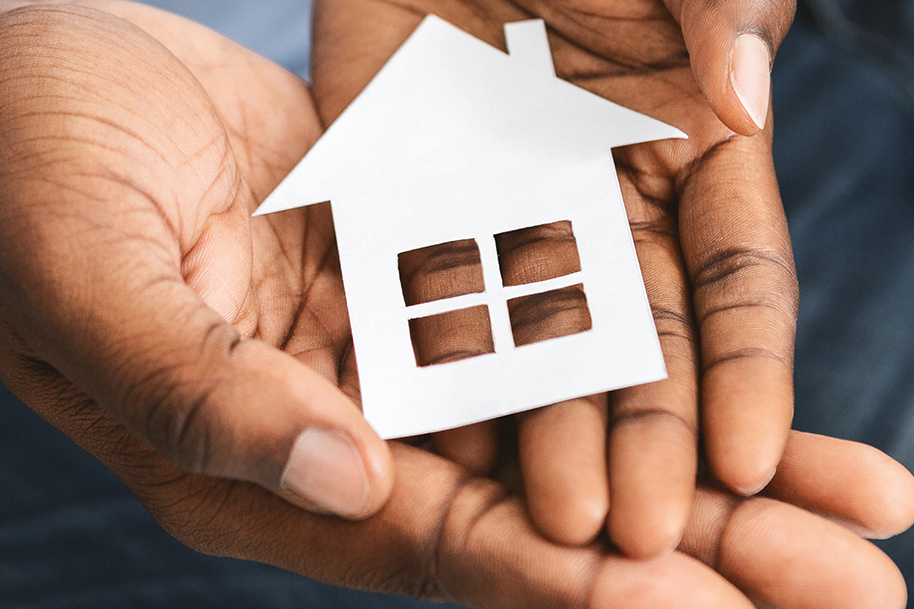
Beyond fairness
The IDSS Initiative on Combatting Systemic Racism explores the role that data, algorithms, and AI can play in advancing racial justice and equity in housing.
For centuries, American ideas and policies around home and land ownership have been framed by racism and colonialism. Through land theft, slavery, segregation, redlining, predatory lending, profiteering landlords, racial covenants, violence, and many forms of discrimination, people of color have been prevented from owning homes, had homes taken from them, and have been robbed of the benefits of fair access to housing and home ownership, from access to other public services (sanitation, water, schools, etc) to wealth accumulation and even longer life expectancies.
Increasingly, computation and big data have become a significant thread in this complex tapestry. Algorithms drive risk assessment in renting and lending, influence property values, and can lead to digital redlining: technology practices that perpetuate and further entrench racial discrimination in housing.
To unravel these systems and replace them with something more fair requires understanding the technology, the history, and the institutions involved. It necessitates collaboration between researchers, data scientists, historians, affected communities, stakeholders, and decision-makers.
Cross-disciplinary collaboration is one of the key features of the Initiative on Combatting Systemic Racism (ICSR), a research initiative started by IDSS. “These issues are very complex. They cut across many different domains, and there are many institutional players involved,” explains Craig Watkins, a Visiting MLK Professor and UT Austin media professor who works with ICSR.
To bring different expertise and perspectives together, ICSR’s Housing team organized ‘Beyond Fairness: Big Data, Racial Justice & Housing,’ a day-long event exploring the intersection of data, algorithms, and AI in relation to housing insecurity, home ownership, and evictions.
The history in the data
There is, as DUSP professor Justin Steil put it, a “legal architecture” to housing discrimination. Resources are distributed unequally through jurisdictions built and zoned to increase inequality. Today, the rate of home ownership among Black families is lower than it was 10 years ago, and trails significantly behind that of white families. The ratio of average wealth between Black and white families has not changed much since the Civil Rights movements of the 1960s, with white families having on average about 8 times the wealth of their Black counterparts.
“This embedding of inequality, of unequal resources in space, obscures some of the causes of socioeconomic inequality and naturalizes it, especially to those who benefit from it,” said Steil. The persisting correlation of poverty, poor housing conditions, and darker skin color — evidence of oppression — can then “reinforce racist ideas about Black people,” added NYU sociologist Jacob Faber.
All this history is embedded in the data, too. “History is something that so often gets left out of our conversations about ethics and technology,” said MIT DUSP professor Catherine D’Ignazio, who co-leads the ICSR Housing team. “Our technology, our data, and our information systems are inflected with and saturated with all the past biases and injustices.”
Housing data can indicate the direct human bias of lenders, appraisers, and realtors, but that data is also evidence of systemic problems in many institutions. “Data reflects entrenched inequality,” said Ben Green, a professor of public policy at the University of Michigan. “In the case of lending, for example, Black loan applicants have less wealth because of historical and current forms of oppression, which means they are at higher risk of default as a result of discrimination.”
“There are aspects like income and location that are indirect bias attributes,” explained Pranay Lohia, a machine learning researcher at Microsoft. “You have removed those direct specific stubs from your data [e.g., race], but there are indirect relations, which are causal relations, because of past dependencies.”
If there’s discrimination in the data, how can algorithms that rely on that data be fair? “The methodology of developing machine learning systems typically fails to account for social context, history, and policy,” said Green. “That leads to a significant gap between the expectations of engineers — that algorithm systems will be fair or will benefit society — and the real-world harms that will result from the actual implementation of these tools in practice.”
Racial capitalism
The cumulative value of Black agricultural land loss in the twentieth century — through legal schemes, racial violence, Jim Crow laws, etc — is estimated by Dania Francis to be around 326 billion dollars. Francis, a professor of economics at UMass Boston, comes from Mississippi, where generations of her family resisted and persisted through this unfair treatment.
Her and her research colleagues arrived at their estimate by examining USDA census of agriculture data, state tax records, and land surveys from 1920-1997, but the amount “is only 3.3% of the estimated $10 trillion dollar racial wealth gap,” said Francis. “It’s still only part of the story… If Black families were not intimidated off their land, they may have used their land to invest in more land. Our estimates do not model this potential counterfactual.”
To quantify the negative impacts of racist policy on Black communities is also to quantify the transfer of wealth to white people. Constraining the housing options of people of color led to suburban white wealth accumulation, said Faber, which leads him to “thinking about discrimination as a technology, a social technology where there’s a scaffolding of a pseudoscientific logic of racial difference.” Racial discrimination in America did not simply disadvantage Black people — Black communities were used as sites for white capital accumulation.
“Under capitalism, you don’t just find systems that discriminate against people along racial divides,” argued Elora Lee Raymond, professor of urban planning at Georgia Tech. “Capitalism is actually a system that creates racial divides, and other divisions between people. That segmentation process at once creates inequality and legitimates it.” This tendency within capitalism to ‘racialize’ groups in order to justify exploitation suggests that racism and capitalism are mutually beneficial, even interdependent — an idea known as racial capitalism.
Contemporary housing systems in the U.S. perpetuate and even worsen this exploitation, and they use contemporary technology to do it. Risk assessment, for example, prioritizes minimizing investor loss when renting and loaning, and entails analyzing increasing amounts of data from criminal records and credit reports to assign risk scores. These scores — like credit scores, which are a relatively recent addition to the US economy — “are highly correlated with and related to systems of historic and current disadvantage along racial lines,” said Eva Rosen, a professor of public policy at Georgetown.
“Credit scores are graded on a curve,” added Raymond. “If everybody makes another on-time payment, we don’t all get a higher credit score. They don’t drift upwards over time or downwards in a crisis. They always have that same shape. And that means that this is a tool for differentially subordinating people and allocating housing to some, but not others. It’s not a tool in which everyone can have access to shelter.”
Justice
In fact, American housing systems routinely push people out of shelter — and both eviction and homelessness disproportionately affect Black and Latino people. Smaller landlords pursue racialized ‘nuisance’ evictions while corporate landlords file for eviction much more often, using the threat of state authority and the police to pressure financially struggling people into debt — or ‘self-eviction’ to avoid having an eviction on their record.
“When we started collecting this information and analyzing it, we had to realize it through the identity of the city,” said Jessica Bellamy, who co-founded the Root Cause Research Center in Louisville, KY. “It’s a plantation state. It’s essentially a Confederate monument.”
“We have to move beyond trying to put neutrality in any of these systems,” said Tawana Petty, a social justice organizer who directs Petty Propolis, a Black women-led artist incubator in Michigan. “It doesn’t exist.”
A computer crunching numbers may seem ‘neutral’ when compared to emotional humans, but the outcomes produced by the technologies employed within the domain of housing are anything but. “If we were able to completely eliminate racial bias in the world tomorrow, we would still see intergenerational inequality in housing opportunity and wealth accumulation because of historical wrongs,” said Faber.
Beyond neutrality and so-called fairness are redress, repair, reparations — in a word, justice. “States and other actors should establish sufficient compensation and reparation schemes for victims of discrimination in housing, especially those belonging to historically marginalized groups,” said Balakrishnan Rajagopal, MIT DUSP professor and UN Special Rapporteur on the Right to Adequate Housing.
What would a more justice-oriented approach to the use and development of algorithms in housing systems look like? “If we hone in on how to design the algorithm, that robs us of the imagination we can have to create completely different systems in society,” said Timnit Gebru, a researcher in AI and ethics and founder of the Distributed Artificial Intelligence Research Institute (DAIR). “If we started from the understanding that everybody deserves a home, the conversation and how we approach these things would be completely different.”