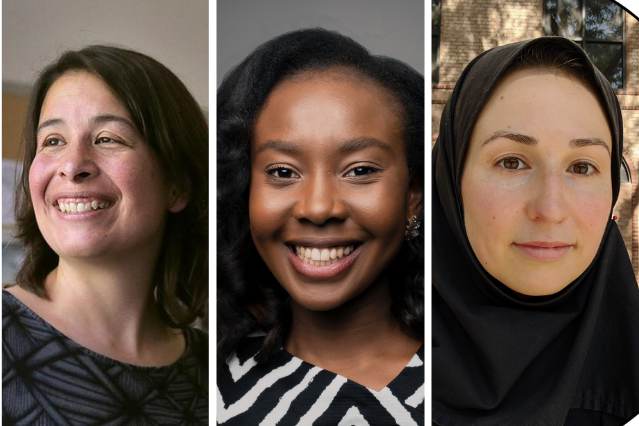
2021 Women in Data Science conference addresses healthcare and bias
from l-r: Anette “Peko” Hosoi, Ehi Nosakhare, Marzyeh Ghassemi
By Greta Friar
The Covid-19 pandemic has been a time of opportunities for and achievements in data science, an interdisciplinary field that analyzes and interprets data to create better models, tools, and solutions to real world problems. At the same time, the pandemic has provided new examples of the risks of biased data science models and misuse of data. The themes of what data science can do for health care and how to address bias and equity within data science were the twin foci of the 2021 Women in Data Science (WiDS) Cambridge conference, held virtually on March 11.
WiDS Cambridge, co-hosted by MIT’s Institute for Data, Systems, and Society (IDSS), the Harvard Institute for Applied Computational Science (IACS), Microsoft Research New England, and as of this year the Broad Institute, is an independently organized regional event tied to the original WiDS conference at Stanford University and intended to celebrate the achievements of women in data science. The conference is also an opportunity for women in data science or looking to get into it to network, discuss cutting edge research, and exchange knowledge and advice.
The first panel of the day, “Machine Learning for Healthcare,” explored how the panelists’ work has helped to advance medical research and tackle issues of bias and inequity in healthcare, such as which patients get represented in predictive healthcare models. The panelists also discussed their concerns about how emergency situations like the pandemic can erode civil liberties, because the massive data gathering and social surveillance used to monitor and control the spread of the virus could set precedents for other more malicious forms of data collection and social control.
Multiple attendees praised the diversity of the panels—speakers came from varied academic fields and industry—for sparking such stimulating conversations. Panelist Marzyeh Ghassemi, who will soon join MIT EECS and IMES as a faculty member, agreed: “The panel was fantastic – machine learning panels rarely have the breadth and depth of experiences required to discuss topics like fairness, health, surveillance and technical scope creep all at once.”
Keynote speaker Anette “Peko” Hosoi, MIT Associate Dean of Engineering and a member of IDSS, described the development of a model to determine how much testing organizations need to do to prevent a Covid-19 outbreak based on factors such as contact tracing and mask-wearing. Hosoi and others within IDSS developed the model, and were then contacted by the NIH’s RADx (Rapid Acceleration of Diagnostics) initiative to turn the model into an online calculator (whentotest.org).
Hosoi hopes that she and other speakers provide women coming into the field with an “existence proof” that women can excel in data science. She sees the goal of conferences like WiDS as being to normalize women in data science, something not yet achieved but getting closer. “WiDS is not just a conference featuring really great women. What stuck with me this year was that this is a conference featuring really great data scientists,” Hosoi said.
The afternoon panel, “Bias and Equity within Data Science,” explored the challenge of building fair and equitable models. The introduction of unintentional assumptions is a persistent problem in data science, one that leads to worse solutions and in certain situations can cause real harm, such as when models reinforce existing stereotypes or under- or overrepresent certain demographics. Panelist and MIT alum Ehi Nosakhare (SM ’13, PhD ’18), now senior data scientist manager at Microsoft, said that an important part of rooting out bias and mitigating harm is improving diversity and representation in data science.
“Imagine if the data scientist working on an algorithm is a member of the community that the algorithm discriminates against, for example a person from a zip code that is constantly denied financial loans. They’re much more likely to notice the bias than someone who does not have that context,” Nosakhare elaborated.
Many conference attendees shared their own research as well. Nearly forty presented posters on the platform Gather.town, a virtual conference space with interactive avatars and video calling, and ten were selected to give pre-recorded lightning talks. A networking session featuring industry booths, again on Gather.town, allowed attendees to speak with representatives from different organizations and explore job opportunities.
Attendees also had an opportunity to get some hands-on experience with data during the Datathon that accompanies the conference. This year’s challenge was to create a model to determine whether patients admitted to the intensive care unit had been diagnosed with a certain type of diabetes. Participants’ data science experience runs the gamut, and the event is meant to be accessible to anyone. To that end, WiDS Cambridge offered a workshop for participants to brush up their skills. Mentors assisted teams and chatted about research experience and career advice.
Weiwei Pan, Harvard IACS research associate, who spearheaded the workshop, says her intention is that everyone leaves feeling like they can do data science. “We don’t lower the ceiling on the difficulty of the challenge; we elevate the floor a little bit so that every team walks away with a basic solution,” Pan says.
WiDS Cambridge concluded with the results of a poll asking attendees where they tuned in from. Thanks to the virtual format, the conference had more than twice as many attendees as usual and they hailed from around the world. The medley of cities that appeared on the screen brought home that women across the globe are busy applying data science tools to important problems, and are eager to connect as they do so.