
- This event has passed.
Other Events
SES Dissertation Defense – Hanwei Li
May 16, 2022 @ 9:00 am - 11:00 am
Hanwei Li (IDSS)
E18-304
Event Navigation
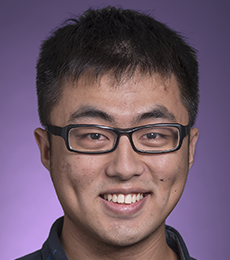
Hanwei Li (IDSS)
E18-304
The emergence of e-commerce business models (such as Airbnb and Amazon) brings opportunities and challenges to their operations. This thesis studies several estimation and optimization problems within the online platform domain, using data-driven approaches in operations management.
The thesis consists of three components. Motivated by the unique setting of Airbnb, in the first work, we consider a game-theoretical setup in which each seller on the platform provides a single-unit product and competes with one another on price. We investigate sellers’ optimal pricing decisions and the platform’s optimal assortment display policy. We find that the platform should display the entire assortment to all the customers when demand is sufficiently high. Moreover, we propose a tabulation algorithm and a mixed-integer programming formulation to effectively solve for the sellers’ and the platform’s optimal decisions. Additionally, we incorporate constraints to guarantee a certain degree of the seller and customer fairness, on both system and individual levels, in the optimal display policy.
The second work is also closely related to marketplaces like Airbnb, where we estimate and optimize the impact of photo layout. We apply Resnet50, a convolutional neural network model, to build two separate, supervised learning models to evaluate the image quality and room types posted by Airbnb hosts. Then, we characterize the overall impacts of photo layout by the room type, photo quality, and display order. To address the estimation challenges in the Airbnb setting, we propose a novel pairwise comparison model to estimate the impact of photo layout consistently. Our estimation results suggest that the cover image has a significantly larger impact than non-cover photos and that a high-quality bedroom cover image leads to the most significant increase in demand. The counterfactual analysis shows the potential impact when adopting the optimal photo layouts.
In the third work, we collaborate with a global online fashion retailer, Zalando, to optimize large-scale price discount decisions. We address Zalando’s local and global business challenges by applying a three-step process. We cluster products into groups that behave similarly and pre-solve the aggregated problem in the first step. In the second step, we decompose the problem using Lagrangian relaxation into a problem for each product (SKU) and provide an efficient way to identify the Lagrange multipliers. Finally, we optimize decisions for individual products addressing local business constraints. For this new approach, which was implemented as part of Zalando’s price discount decision process, we provide results from offline tests and field experiments to demonstrate its benefit.
David Simchi-Levi (chair, supervisor), Stephen Graves, Víctor Martínez de Albéniz (IESE Business School)
Hybrid event. To attend virtually, please contact the IDSS Academic Office (idss_academic_office@mit.edu) for connection information.
MIT Institute for Data, Systems, and Society
Massachusetts Institute of Technology
77 Massachusetts Avenue
Cambridge, MA 02139-4307
617-253-1764