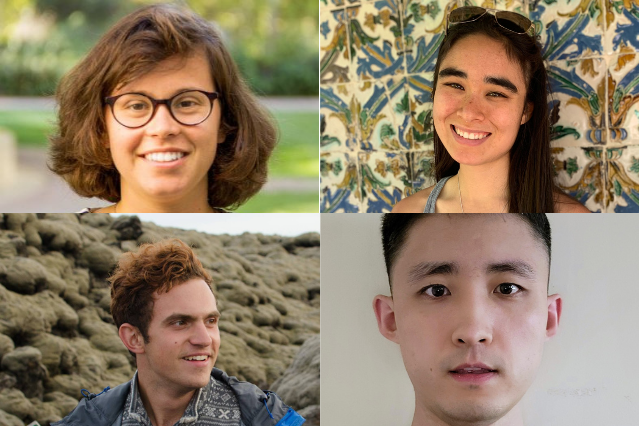
IDSS MicroMasters Teaching Assistants
As part of its mission to advance education in data analysis, IDSS faculty developed the online MicroMasters Program in Statistics and Data Science (MM SDS), which boasts thousands of learners from around the world. To enhance the experience of learners, including those engaged through an IDSS strategic partnership in education, IDSS TAs provide synchronous support to each MM SDS course. IDSS TAs are either PhD students in the Social and Engineering Systems (SES) program, or postdoctoral scholars on the IDSS research staff.
“The teaching assistants for the MicroMasters program are carefully selected based on their expertise, teaching experience and philosophy,” says Karene Chu, Assistant Director of Education of the MM SDS at IDSS. “They are passionate about designing and improving the theoretical exercises and hands-on projects that supplement the online courses. They contribute their deep knowledge and are compassionate when communicating with learners with widely varying backgrounds from around the globe. Their continual support to the learners is crucial in making the online program a truly educational experience.”
“We’ve recently made being an MM SDS TA a requirement for the SES program,” says Devavrat Shah, an EECS and IDSS professor who directs the MM SDS program. “This is rewarding work that reinforces statistical concepts and develops important skills for IDSS students. The workload balances well with their courses and research, and it puts our students at the forefront of a major IDSS effort to advance education in statistics and data science.”
Meet the TAs
Marie-Laure Charpignon
Marie-Laure Charpignon is a PhD student at IDSS, conducting research at the Laboratory for Information and Decision Systems (LIDS) and the Harvard-MIT Department of Health, Sciences, and Technology (HST).
Her research interests include causal inference, agent-based modeling, and text analysis, with applications in public health. Her focus is on the development of competing risk causal inference approaches to emulate target clinical trials for Alzheimer’s disease, using observational data from Electronic Health Records in the US and UK.
How has being an IDSS education partner MicroMasters TA complemented your SES experience?
Since I joined IDSS as a doctoral student, I have had the opportunity to assist with the Statistics, Computation, and Applications course both in-person and online. On campus, I enjoy interacting with students during recitations, office hours, and project brainstorming sessions. Teaching the fundamentals of data science as well as coding tips for large-scale data analysis, algorithm implementation, and visualization is a lot of fun! Furthermore, it helps me hone my theoretical skills by motivating me to not only understand and apply them in my own research concepts, which range from time series to network science, but also communicate effectively to a wide audience of undergraduates, graduate students, and professionals. Because I co-taught the class with other instructors, including TAs and well-established professors, I also learned from my peers and from senior educators about teaching techniques and thereby expanded my pedagogical toolbox!
As Karene gave me the opportunity to contribute to the design of the first version of the online course, we had to think creatively about incentivizing on-platform student engagement and frameworks for sharing peer-to-peer feedback. As a PhD student in SES, I am building upon my own experience as a student at MIT to help improve the learning experience of others, whether on campus or online.
What’s a common learner challenge and how do you approach it?
Data science is a truly interdisciplinary field! Being a great data scientist requires a combination of (1) solid foundations in applied mathematics, including linear algebra, functional analysis, and optimization, (2) an appetite for complex systems modeling, as well as (3) domain knowledge and creativity. A common learner challenge is the ability to translate a real-world problem into its mathematical formulation, further design and implement an algorithmic solution for it, and critically interpret the results.
Learners in the MM SDS program develop skills like how to formulate research questions of/on their own, how to collect relevant data, and how to derive insights that domain experts can take action on. For learners to gain familiarity with the data science process, I like to start with illustrations in low dimension before abstracting away to the more general, potentially high-dimensional scenario.
What do you like most about being an MM SDS TA?
An important part of being an MM SDS TA is contributing to the design of a strong curriculum tailored to online learners. Along with experienced educators and instructors in mathematical sciences, I have helped define learning outcomes for each module and refine them over the past two years as the needs and practices of the research and industry fields evolve. For example, the involvement of professors Uhler and Jegelka with the Schwarzman College of Computing’s Social and Ethical Responsibilities of Computing (SERC) group encouraged the teaching team to integrate data ethics considerations as part of the network science module. In practice, this involved prompting learners to think about issues related to criminal networks and policing. Interestingly, learners reacted positively and proactively to this shift, aimed at emphasizing the social implications of computational research.
Through guest lectures, learners also gain exposure to state-of-the-art research, while being at the forefront of pressing topics like data governance, privacy, and ethics. Rich follow-up discussions emerge, sometimes even leading to longer-term research collaborations!
How are you able to apply your experience with residential courses to the recitation sessions within MicroMasters courses?
The MM SDS courses taught me to think about the set of questions to ask in a live interaction context to help a student strengthen their skillset. Personalized learning in the classroom is more difficult than in online settings, where there are more tutoring opportunities. Being very intentional about the learning objectives for each module component helped me enhance the quality of my in-person recitations, prompting the audience with active learning questions that catalyze analogies between mathematical concepts and real-world problems.
Michelle Vaccaro
An SES PhD student within IDSS, Michelle focuses on human-AI decision-systems, addressing questions such as: How can we model hybrid decision-making at the individual and collective level? How can algorithms and interfaces complement people’s natural intelligence? And how do they affect individual and group decisions and experiences in complex systems?
Prior to joining MIT, Michelle worked at Goldman Sachs in foreign exchange strategy and structuring, and earned a Bachelor’s degree in Computer Science at Harvard.
How has being an IDSS education partner MicroMasters TA complemented your SES experience?
Since many learners in the program work full time jobs, they helped me better understand the potential to use more rigorous statistics and data science across so many industries. I hope that my research contributes to understanding, modeling, and improving decisions in their domains of expertise!
What’s a common learner challenge and how do you approach it?
A large proportion of learners in the course hadn’t taken calculus since high school, so they found the units requiring differentiation and integration somewhat daunting at first. In an attempt to help, we created additional review resources that taught these concepts assuming no prior knowledge. By the end of the course, students were integrating by parts with ease!
What do you like most about being an MM SDS TA?
I love working with the MicroMasters program because it allows me to interact with incredibly diverse learners, and to gain insights into the applications of statistics and data science in a wide variety of domains.
How has the course material in MM influence/benefitted current learners?
Many learners applied the material from the course almost immediately in their current jobs. For example, some learners in 6.431x started to use the Bayesian models we studied in domains such as manufacturing and finance!
Max Vilgalis
Max’s academic career has always been motivated by climate change. As an undergrad, he hoped to develop better renewable energy technology, so he studied electrical engineering and worked in energy systems. However, through internships in research, industry, and government, he came to realize that energy and environmental challenges cannot be addressed by new technologies alone. Max came to MIT to learn to use quantitative analysis to inform environmental policy decisions, and is now an SES PhD student at IDSS. He studies adaptation to climate change using nonparametric statistics and structural econometrics – that is, he’s interested in using evidence from people and firms’ decisions to reveal how much they are responding to climate changes such as increasing fire risk and extreme heat.
As a TA, Max has worked with special cohorts of learners in Uruguay through an IDSS strategic partnership in education.
How has being an IDSS education partner MicroMasters TA complemented your SES experience?
Teaching with the MicroMasters has been an excellent complement to my other role as a researcher. As a researcher, your goal is to push the boundary of what is known. It can be exciting, but it can also be frustrating when few ideas work and you feel like you don’t know anything. Teaching forces me to reflect on the fact that I’ve learned a lot, and that I am already able to help people even if my projects aren’t exactly where I’d want them to be. Teaching also forces me to think about how to effectively share concepts and ideas, which is obviously essential to being a good researcher.
What’s a common learner challenge and how do you approach it?
I think especially in statistics, many learners feel a disconnect between learning the theory and learning the practice. I think the best way to overcome this is to mix theory to introduce concepts with active practice implementing them. Most of the lectures on the MicroMasters courses already do a great job of this, and it’s something I try to emulate while conducting recitations.
What do you like most about being an MM SDS TA?
It’s a great opportunity to work with learners from outside MIT, who bring a bit more life experience into the classroom. I’m so in awe of our learners for having the curiosity and drive to take these classes in addition to their full work schedules. It’s a privilege to help support that commitment to learning in whatever small way I can.
Silun Zhang
Silun is a Postdoctoral Associate with IDSS and LIDS working in complex large-scale systems, distributed control, and privacy in multi-party computation. His research is to solve the problems of control, optimization, as well as learning when the data or/and computation power required in the problems is distributed across networks. Before he came to MIT, he obtained a PhD in applied mathematics at KTH Royal Institute of Technology, Sweden. He has been involved with the development of course 6.419x — Data Analysis: Statistical Modeling and Computation in Applications.
How has being an IDSS MicroMasters TA informed your work?
The Data Analysis course I am teaching is quite exclusive in two aspects. First, it involves a wide range of data analysis techniques in modern statistical learning, spanning from basic probability, statistics, time series, to Gaussian processes. The course manages to organize all these diverse materials in a consistent and concise manner to build a solid theoretical foundation for each learner. Second, the course associates the theories tightly with realistic problems. There are a large number of exercises, quizzes, and projects coming from real applications that are continuously added to the course, such as social networks, economic trends, and genomic analysis.
Therefore, I recognize teaching and developing this course as a unique opportunity to streamline my own knowledge hierarchy related to data science and statistical learning. From this process, several ideas about developing decentralized algorithms for the tasks of statistical inference have been raised for my future research. In addition, the various examples based on real datasets given in the course also enable me to link the abstract math problems I am working on with different real applications. As an applied mathematician, such a link is regarded as very valuable.
What’s a common learner challenge and how do you approach it?
The course 6.419x contains a large number of learners that are coming from diverse academic experiences and occupational backgrounds. Some learners, for example, may lack theoretical knowledge in a specific area, or have limited coding experience in Python and a popular library. These hurdles often make the learners feel challenged to catch up with the course pace and lag behind other attendees. To address this issue, the course team and I collected common confusions of these learners from the discussion forum, and then offered supplemental, supportive content covering prerequisite knowledge, recapping exercises, and referencing textbooks and articles from the course. We also added extra coding demonstrations in the recitation sessions. In such a way, the whole course is presented in a more scaffolded and easy-to-follow fashion.
What do you like most about being an MM SDS TA?
I am always thinking that a major pattern of being a scholar is iterating between learning new pieces of knowledge and finding both illustrative and insightful languages to deliver them to the audience. Therefore, I recognize being an MM SDS TA as a perfect opportunity to develop my teaching ability, which is a necessary step towards becoming a qualified scholar. Thanks to the diversity of learners in this course, I have learned how to develop and organize lectures for general audiences. This is a precious experience for my future career.
In addition, I deeply enjoy the collaboration and interactions with the course team. We together collected and analyzed the learner’s feedback in weekly meetings, and added new materials for better course delivery. Everyone in the team is dedicated to providing quality support for learners.